“Perfect is the enemy of good,” the old saying goes, and nowhere is that truer than in today’s race to adopt AI in business. Consider two companies: Pristine Manufacturing Corp. spends years scrubbing its data and formulating strict AI policies before ever launching a single AI project. Upstart Manufacturing, Inc, a competitor, starts small by deploying a chatbot pilot on their messy FAQ data and using the learnings to gradually improve. Six months down the line, Upstart has learned about their users’ intents and expectations, while Pristine is polishing its “AI Framework” and falling behind. This scenario may be a parable, but it reflects a real trend: in the era of AI, progress often beats perfection.
What’s Slowing Down Enterprises in AI Adoption
Many organizations hesitate to dive into AI for understandable reasons. Whether it is technology that can be daunting, legal requirements that are unclear and evolving, or simply the high reputation, ethics and accuracy stakes, up to 75% of large enterprises have admitted that they’re delaying AI initiatives due to these concerns.
Actionable Steps to Embrace AI Now
Transitioning from recognizing the pitfalls of perfectionism to taking concrete steps toward AI adoption is crucial. Here are practical actions your organization can undertake:
- Define Clear Objectives: Identify specific business challenges that AI can address, focusing on business value creation, implementation effort, solution maturity and risk.
- Start with Pilot Projects: Initiate small-scale AI projects to test feasibility and gather insights. This approach allows for manageable risk and provides valuable learnings that can inform larger-scale implementations. Develop intra-organization forums for insights sharing.
- Assess Data Readiness & Define Clear Guidelines: Evaluate the quality and accessibility of your existing data, defining specific and objective concerns and risks. While perfection isn’t necessary, understanding your data landscape and its environment helps in selecting appropriate AI tools and identifying areas for improvement (or limiting areas where applying AI would lead of adverse outcomes).
- Invest in Training and Resources: Equip your team with the necessary skills and knowledge to work with AI technologies. This investment fosters a culture of innovation and ensures that your workforce can effectively leverage AI tools.
- Establish Ethical Guidelines: Develop a framework to address ethical considerations in AI deployment, such as data privacy and bias. Clear guidelines help in building trust and ensuring responsible AI use.
- Monitor and Iterate: Continuously assess the performance of AI initiatives and be prepared to make adjustments. Embracing a cycle of monitoring and iteration leads to sustained improvement and alignment with business objectives.
Prioritizing Clarity and Flexibility
Enterprises crave clear guidelines, perfect datasets, and assured outcomes. But here’s the reality: if you wait for everything to be perfect, you’ll wait forever, and, perhaps more importantly, you will not benefit from learnings and data gleaned from your early-stage use cases. Meanwhile, more agile competitors will be reaping the rewards of early AI adoption (or, perhaps, shutting down their unsuccessful pilots with learnings). The good news is that you don’t need a pristine environment or ironclad rulebook to get started. By prioritizing progress over perfection, and coupling it with some common-sense risk management, you can start unlocking AI’s value today, not someday.
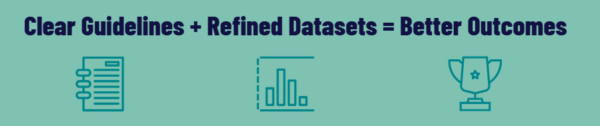
About the Author
Derek Perry is the Chief Technology Officer at Sparq, leading the company’s AI-First strategy and driving innovation through the development of strategic, AI-centric service offerings.
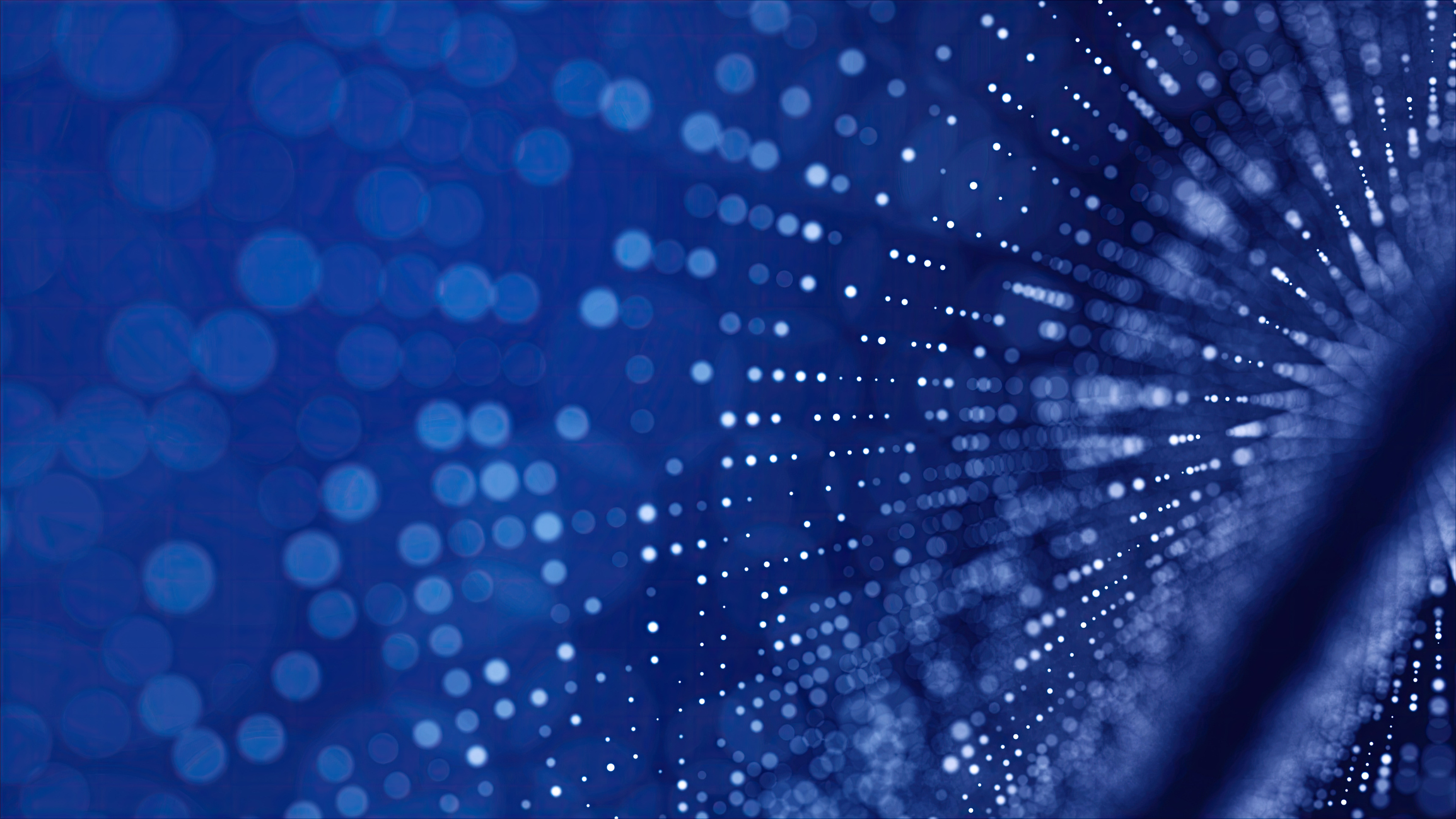
Analysis Paralysis in AI Adoption
Learn why endless discussions and the relentless pursuit of flawless data are actually costing you valuable time, insights, and competitive advantage – just like it did for giants like Kodak and Blockbuster.
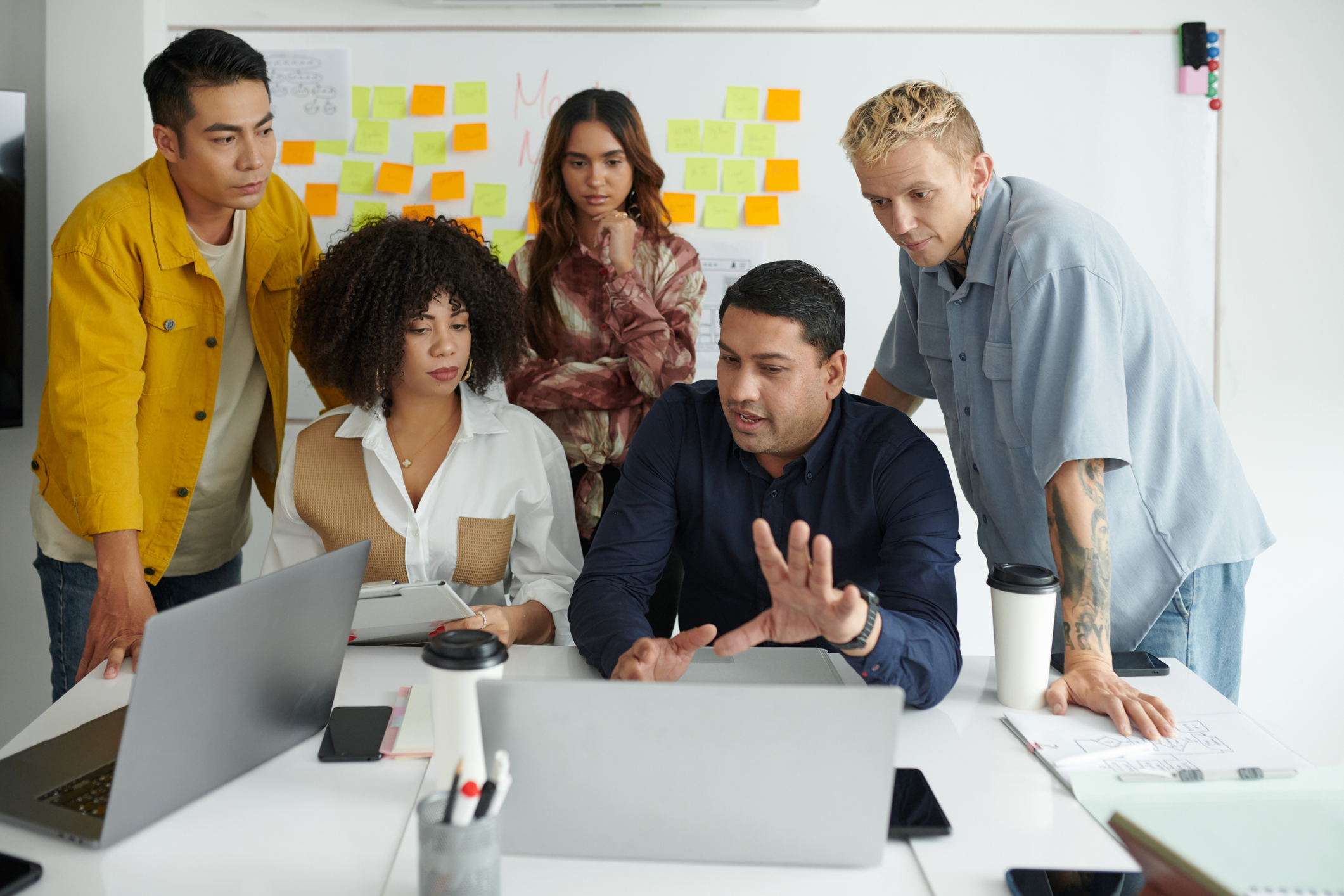
Don’t Take Product Out of the Equation: How to Nail Your AI Implementation
AI isn't just about the technology, it's about solving real problems and delivering real value. One way to do that is to keep product at the forefront during your AI implementation. Learn more about why having a product-first mindset is so important in this article by Principal Product Strategist Heather Harris.
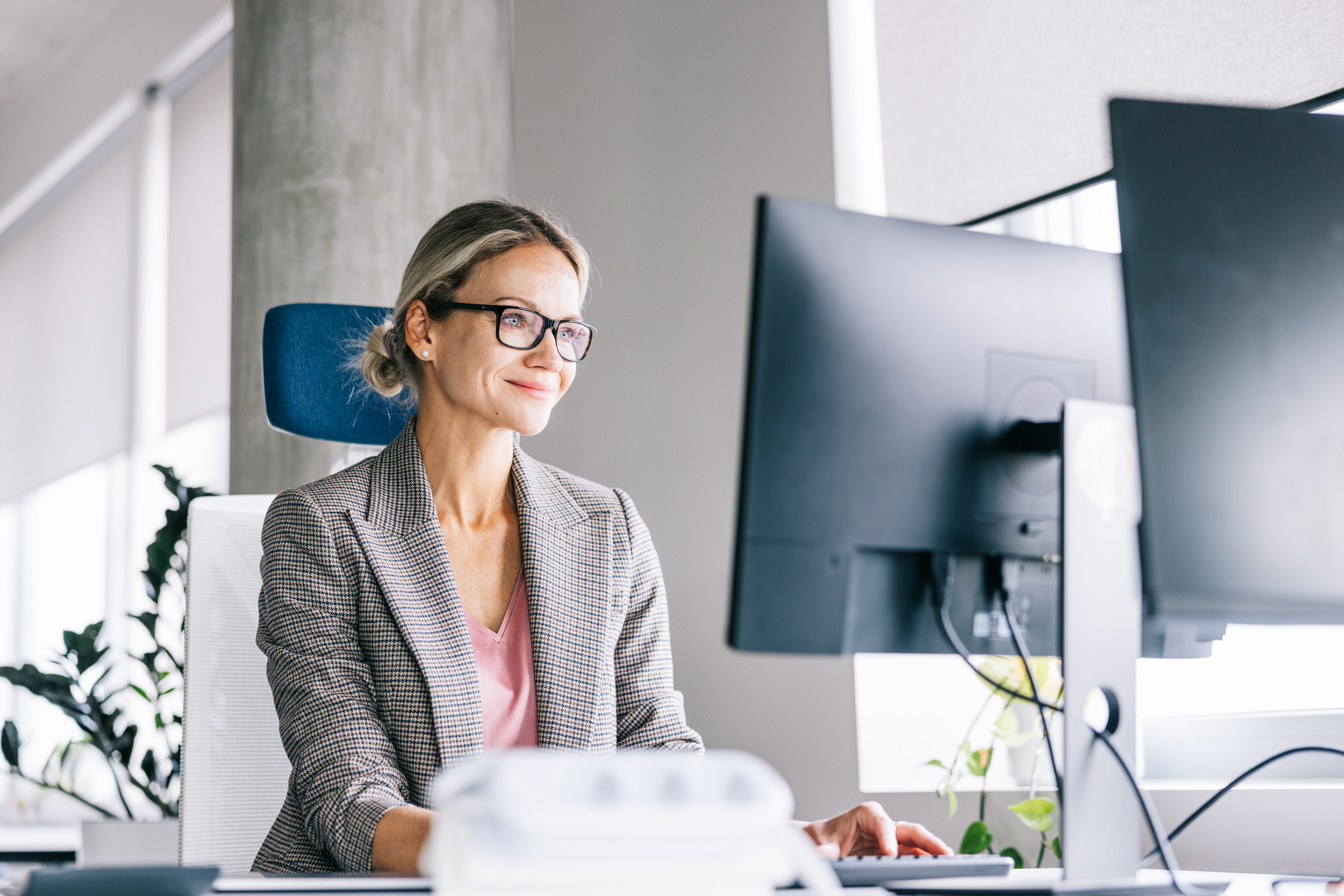
Navigating AI in Banking and Financial Services: A Risk-Based Rebellion for Leaders
Every shiny AI use case in regulated industries has a shadow: governance, compliance, model risk, ethics, bias, explainability, cyberattack vectors and more. It's not that organizations and leaders don’t want AI, it’s that they’re paralyzed by the political, regulatory, and operational realities of deploying it. Sparq's Chief Technology Officer Derek Perry and VP, BFSI Industry Leader Rob Murray argue we need to change that. Check out this article to learn how to actually ship production AI use cases in regulated environments.
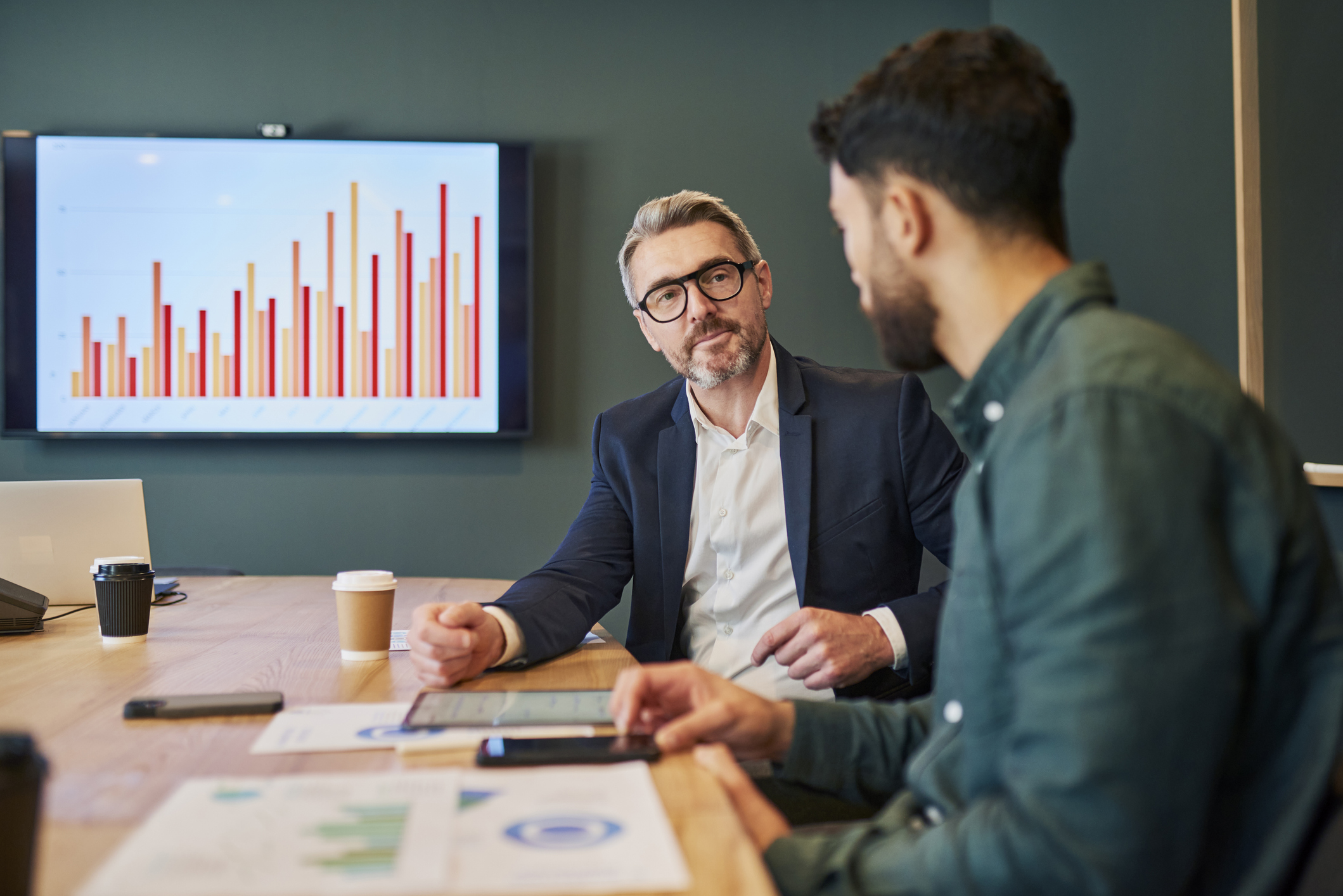
Five Important Questions to Ask Before Starting Your AI Implementation
Creating a lasting impact with AI requires more than just technical output. In this article by Principal Product Strategist Heather Harris, learn five questions to ask before starting an AI implementation so it can deliver long-term business value.