“Never invest in a business you cannot understand.”
Replace “business” with “AI” in this quote from Warren Buffett and you’ve got the modern dilemma for leaders in regulated industries.
In banking and financial services, AI isn’t just hype anymore, it has arrived (albeit not uniformly distributed). It’s underwriting loans in milliseconds, preventing fraud before it happens, and generating custom investment advice at scale. But let’s be honest: while startups and fintechs sprint ahead, too many traditional firms are still caught in an eternal strategy loop.
Why?
Because in regulated industries, every shiny AI use case has a shadow: governance, compliance, model risk, ethics, bias, explainability, cyberattack vectors and more. It’s not that organizations and leaders don’t want AI, it’s that they’re paralyzed by the political, regulatory, and operational realities of deploying it.
Let’s change that. Let’s talk about how to actually ship production AI use cases in these environments.
The False Choice Between Risk and Velocity
If you’re a line-of-business exec trying to push forward an AI idea, let’s say smarter credit scoring or real-time fraud detection or even more straightforward cases like document processing, chances are you’ve heard this from your risk team:
“We can’t move forward until we’ve done a full regulatory impact analysis and validation of the inner workings of the AI model with the legal team.”
Translation: good luck, see you in 18 months.
But here’s the kicker: doing nothing is not, in fact, safer. In fact, Gartner warns that failing to adopt AI now could leave firms operationally inefficient, non-competitive, and more vulnerable to both business and compliance threats.
So what’s the move? A practical, political, risk-based approach that helps AI projects not just start, but deliver value in production and evolve.
A Playbook for Getting AI Done
Start Where the Risk is Lower
Not all AI use cases are created equal. If you’re trying to launch your first project in underwriting or claims decisioning, you’re walking into a regulatory buzzsaw. Instead, start with:
- Document automation
- Internal knowledge assistants
- Personalization engines for marketing segmentation
These are low-risk, high-ROI use cases that don’t trigger the same legal and audit speedbumps.
Pro tip: Using commonly accepted industry standard approaches for risk management will help, such as
- Gartner’s TRiSM model (Trust, Risk and Security Management)
- NIST AI Risk Management Framework (AI RMF 1.0)
Stack Your Allies
No one builds production-grade AI alone. You need:
- Compliance to align innovation with regulatory expectations and develop guardrails
- IT/Security to deploy in the right architecture
- Product & Ops to pressure-test the ROI
- Executive sponsors to shield you from the political crossfire
Gartner suggests forming a cross-functional AI governance board, but it can’t be just a committee that meets and nods or denies risk-managed progress. It needs to function as a tiger team with real decision rights, empowered to unblock progress and own outcomes (and be measured as such!).
Proof of Concept ≠ Science Fair
Your POC must be designed like a Trojan horse. It should:
- Use anonymized or synthetic data to stay compliant
- Be scoped tightly (30-60 days max)
- Show clear KPI lift tied to dollars, hours saved, or risk reduction
- Include a path to production from day one
If your POC doesn’t have a deployment plan, it’s just academic cosplay.
Win the Model Governance Game
In BFSI, it’s not enough for a model to work, it has to explain itself. Auditors want:
- Model documentation
- Decision trees or Shapley value interpretations
- Change logs
- Ongoing monitoring for drift
Treat your models like you would financial instruments; track them like they can bite you. Because they can.
Scale Responsibly – But Actually Scale
Once your MVP is in place and delivering, don’t let it stall. Build toward production with:
- Cloud-native pipelines that automate testing, monitoring, and rollback
- A ModelOps layer that separates experiments from production-grade models
- Continuous retraining workflows based on real-world feedback
- Vendor accountability frameworks if you’re integrating 3rd party models
Don’t forget to budget for prompt tuning, retraining, and regulatory remediation. This is a marathon, not a launch party.
But What About the Regulators?
Good question. U.S., EU, and APAC regulators are moving fast, often faster than your IT team. The EU AI Act, China’s tightening controls, and state-level legislation in the U.S. (California, Colorado, Texas) all require proactive positioning.
Don’t wait to be told what’s required. Instead:
- Engage regulators early, especially if your use case involves consumer decisions
- Maintain an auditable trail of your model training and performance
- Build regulatory simulation environments to test compliance before going live and to tabletop scenarios
If you’re not treating regulators like stakeholders, you’re already behind.
Closing Thought: Be the One Who Delivers
Let’s face it: in BFSI, AI doesn’t get killed by tech debt. It gets killed by organizational fear, uncertainty and doubt.
If you want to be the leader who moves the needle, you need to master the art of AI diplomacy: balancing speed, ethics, business value, and regulatory strategy.
Or as Buffett might’ve put it today: “Never deploy an AI model you can’t explain to a boardroom, defend to a regulator, and improve with your team.”
We don’t need more pilots. We need more applied AI solutions that are governed, trusted, and delivering ROI.
Be the one who ships.
Learn more about how Sparq helps banking and financial services organizations navigate AI.
About the Authors
Derek Perry is the Chief Technology Officer at Sparq, leading the company’s AI-First strategy and driving innovation through the development of strategic, AI-centric service offerings.
Rob Murray is the Vice President, BFSI Industry Leader responsible for driving the banking, financial services & insurance (BFSI) vertical growth. He leads the development, implementation and execution of the vertical’s Go-to-Market strategy and the BFSI sales organization.
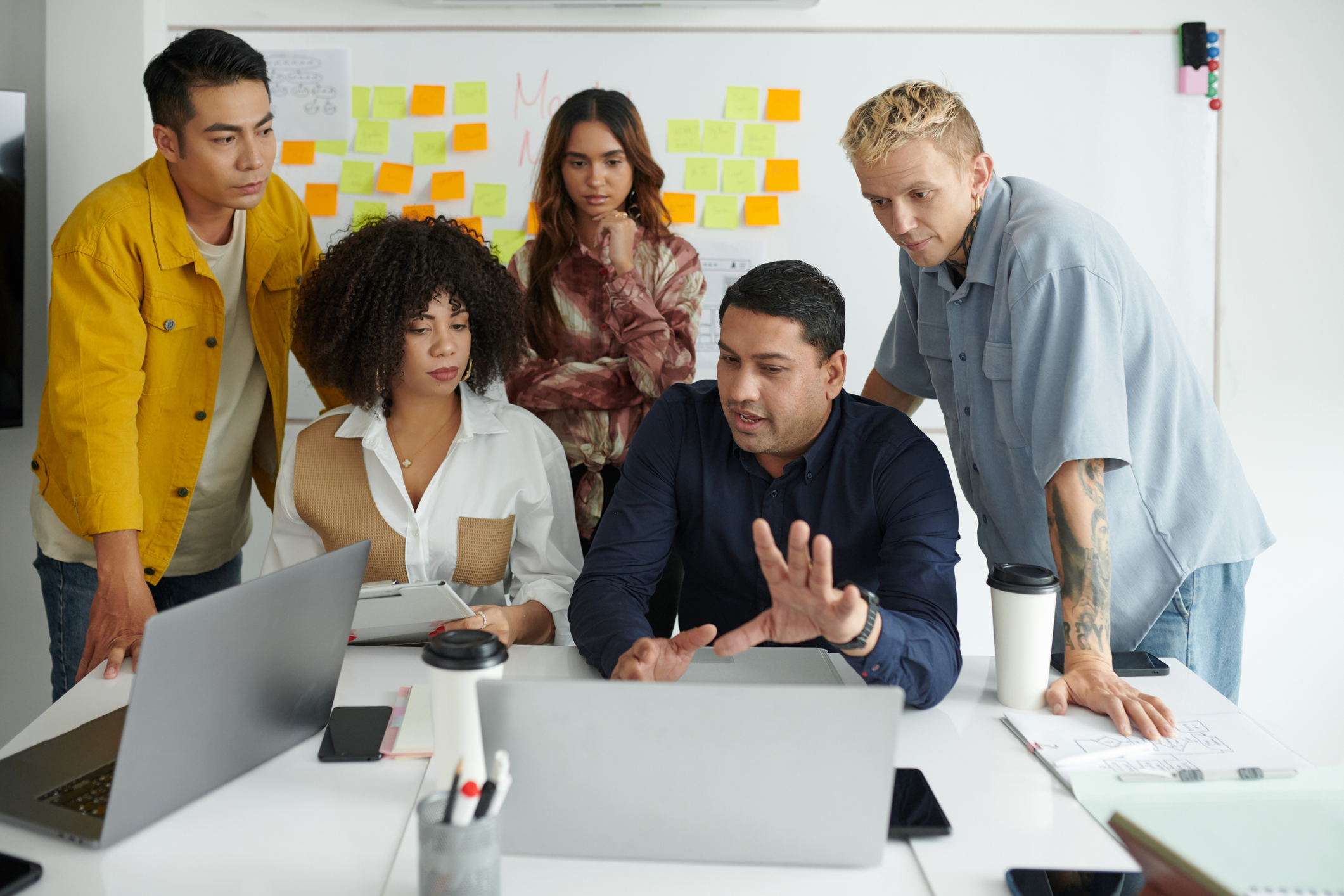
Don’t Take Product Out of the Equation: How to Nail Your AI Implementation
AI isn't just about the technology, it's about solving real problems and delivering real value. One way to do that is to keep product at the forefront during your AI implementation. Learn more about why having a product-first mindset is so important in this article by Principal Product Strategist Heather Harris.
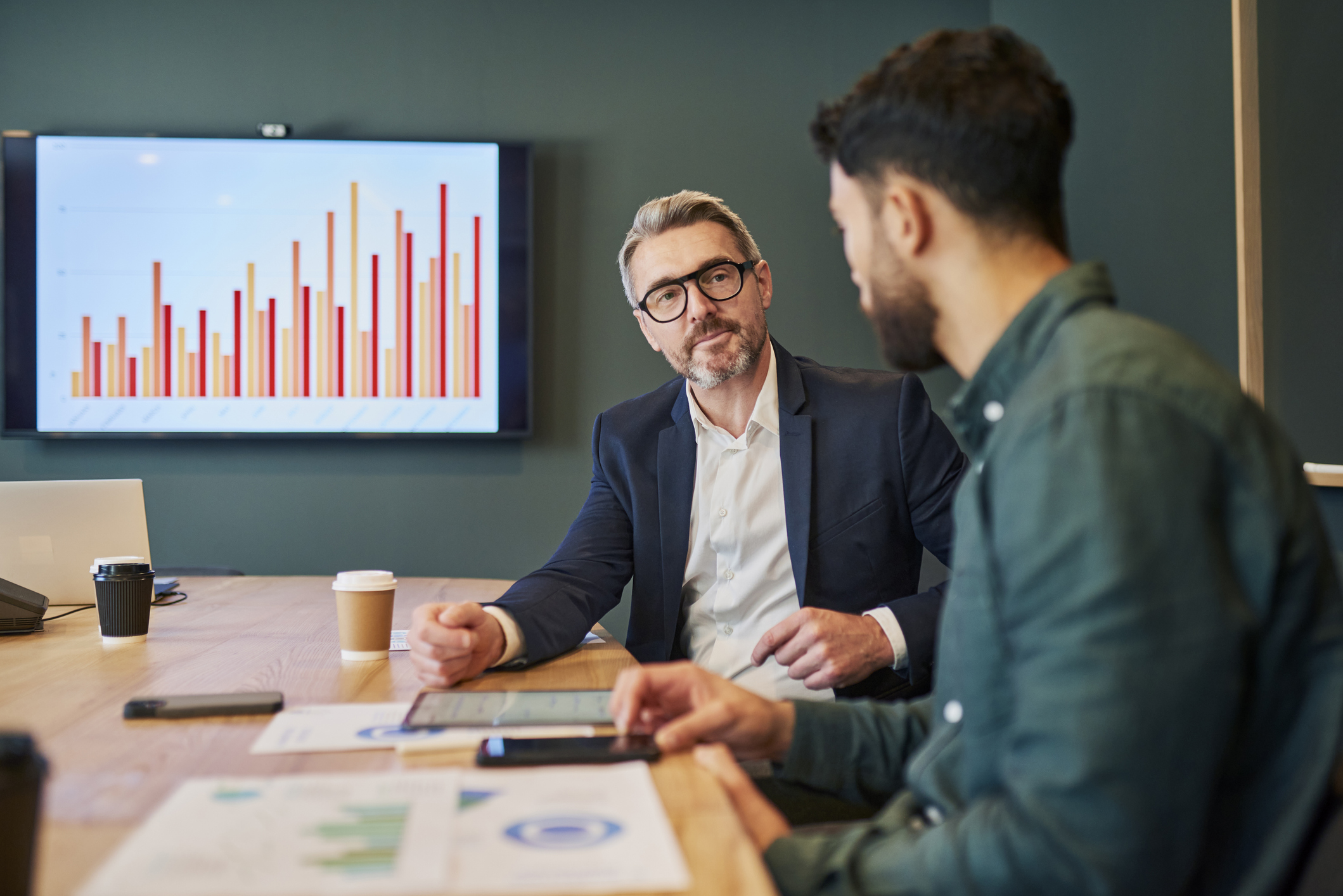
Five Important Questions to Ask Before Your AI Implementation
Creating a lasting impact with AI requires more than just technical output. In this article by Principal Product Strategist Heather Harris, learn five questions to ask before starting an AI implementation so it can deliver long-term business value.
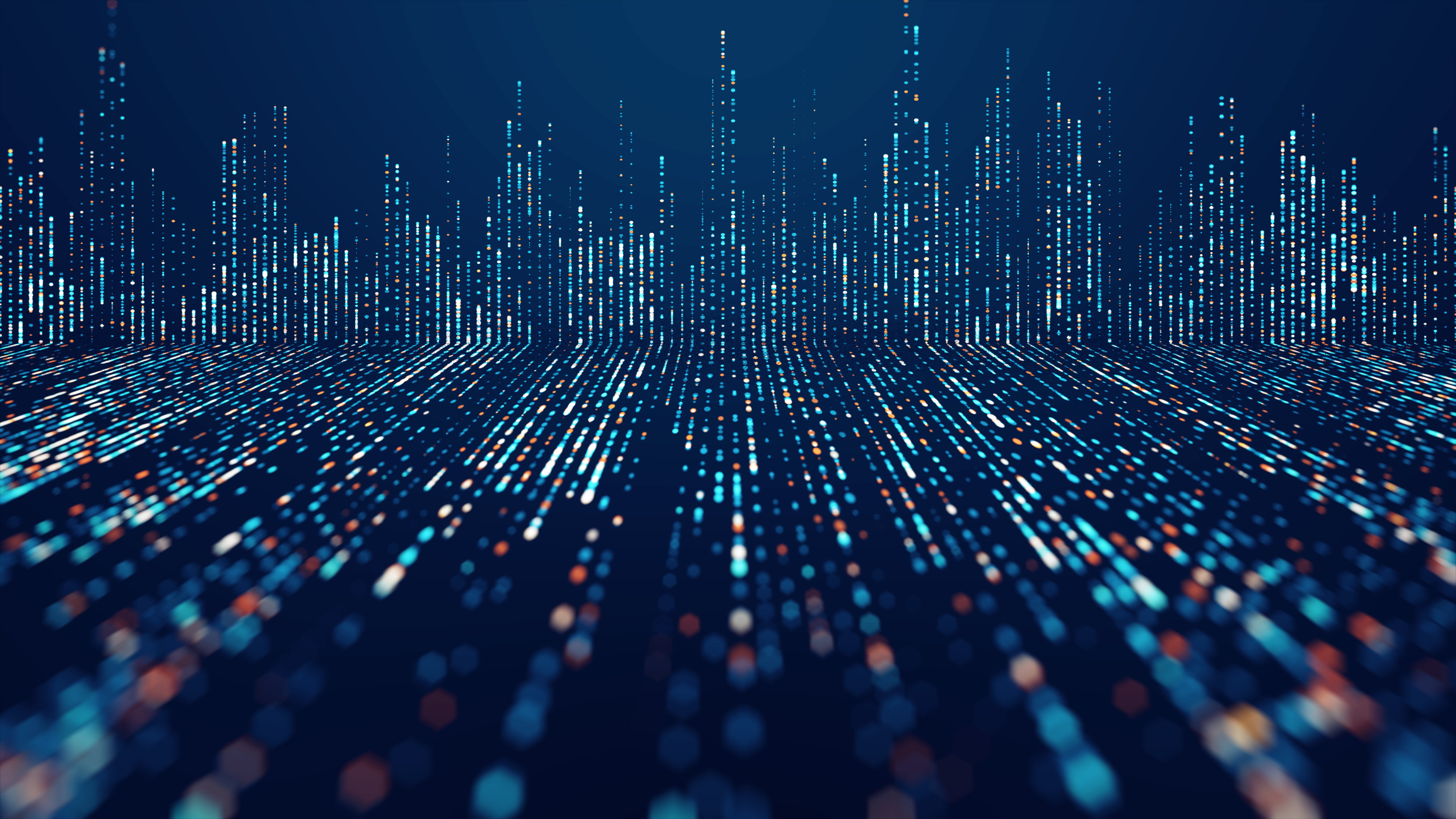
Progress Over Perfection: How to Embrace AI Now (Without Waiting for Perfect Data)
“Perfect is the enemy of good,” the old saying goes, and nowhere is that truer than in today’s race to adopt AI in business. In this article, Sparq CTO Derek Perry shares actionable steps for organizations to embrace AI now (without waiting for perfect data).
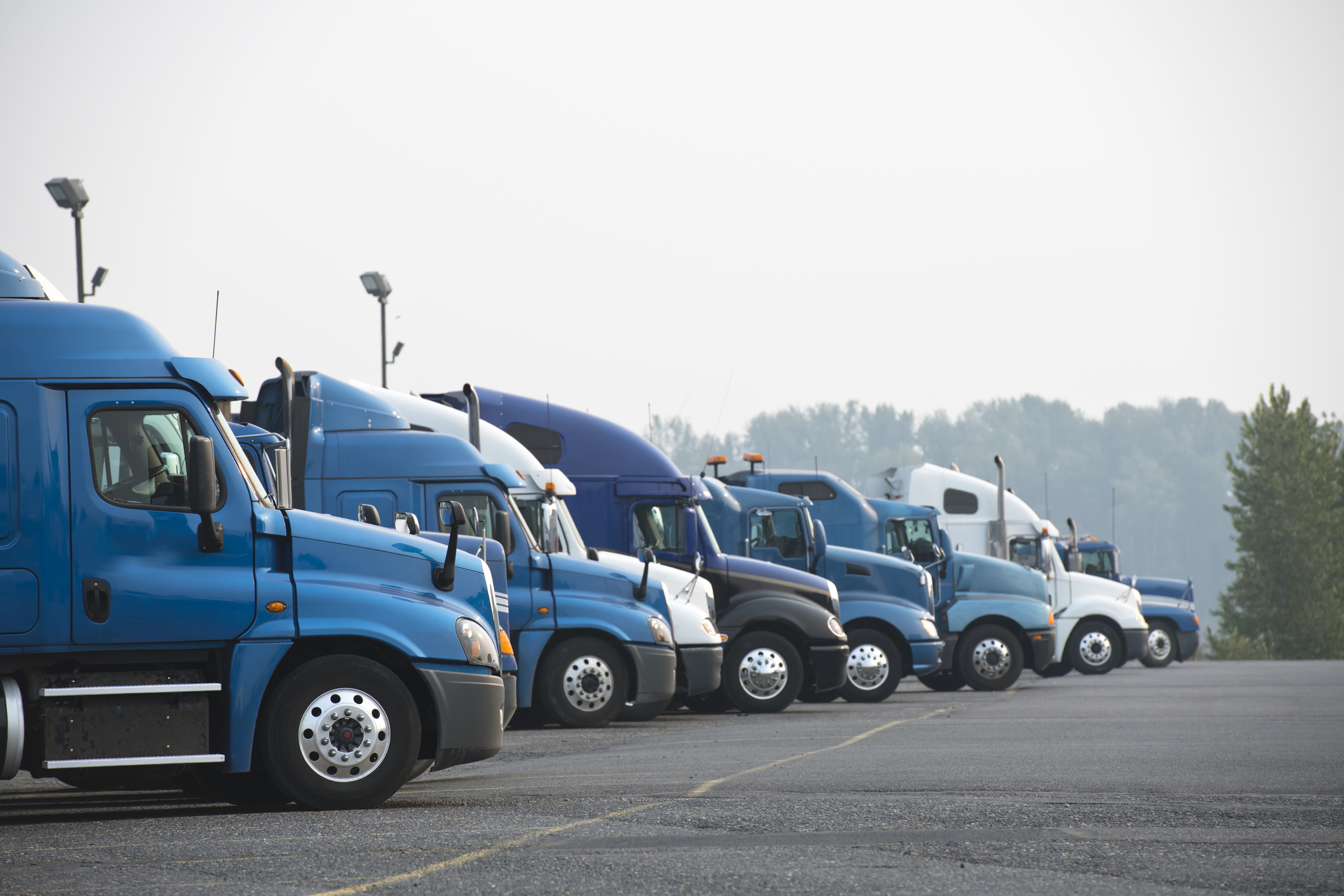
Key Takeaways from TAG’s “Connected Fleet” Event
Sparq was honored to sponsor the Technology Association of Georgia's “Connected Fleet: The Future of Transportation Technology and Logistics Optimization” event, which featured an accomplished panel of technology leaders in transportation & logistics. The panel had an insightful discussion around what's shaping the future of innovation in transportation & logistics. Read on for some key takeaways from the event.