“The maxim ‘Nothing avails but perfection’ may be spelt shorter: ‘Paralysis.'” is famously attributed to Winston Churchill, reflecting his action-oriented orientation.
Does this sound familiar? Your team has been talking about implementing AI for two years. Maybe it’s automating customer support with a chatbot, or using machine learning to streamline supply chain forecasts. But every meeting ends with, “We’re not ready yet.” Perhaps the customer data might have errors, or you’re waiting on a new data governance policy. The result? Nothing moves forward. This is analysis paralysis. Of course, there are numerous famous cases where analysis paralysis impacted a business negatively (Kodak and digital film; Blockbuster and streaming video).
Concerns are necessary and healthy, but when they completely stall progress, they become a problem in themselves. Instead of framing concerns in general language, specifically stating concerns or risks is more effective. Rather than “our data is bad“, think “our data has a gap due to a telemetry error from August 2024 to June 2024 and our data prior to January 2021 does not have the same attributes for customer records”. This specificity allows for mitigations to be put into place. Concerns with specificity combined with organizational policies, procedures and affinities can then emerge as clear guidelines.
The cost of waiting can be huge, with not only an impact in the present, but a lack of learning and insight and less data to reinforce or augment further AI products or decision making.
What’s often misunderstood is that AI adoption is not an all-or-nothing leap. You don’t have to implement a mission-critical, fully autonomous AI system on day one. In fact, you shouldn’t. The smarter approach is to start with a small pilot, something manageable that addresses a real business need. The second part is critical; there must be utility and value that would be derived from using this model. This could be as simple as an AI tool to categorize support tickets, or a basic predictive model to flag inventory restock needs. Keep the scope limited. The goal is to get a win on the board and gain practical experience.
Leading AI practitioner Andrew Ng advises business leaders that it’s “better to start small than to start too large”. He’s seen more companies fail from giant moonshot AI projects than from modest pilots. At Google, Ng’s team first applied deep learning to improve voice recognition (a contained project) before trying to tackle bigger products. That quick success got everyone on board for larger AI investments. In this way, voice recognition was both a pilot to learn from as well as a foundational component of downstream AI innovation, used within a multitude of products and applications.
The takeaway: Don’t let “we’re not ready” stop you from getting ready. Identify one area where AI could help and where imperfect data or planning won’t do irreparable harm. Consider mitigation strategies. Then just start. Think of it as an experiment with upside – even if it doesn’t fully work, you’ll learn why and can iterate. Progress isn’t about recklessness; it’s about consistent, forward movement, however measured the steps.
Learn how Sparq can help you get started on your AI journey.
About the Author
Derek Perry is the Chief Technology Officer at Sparq, leading the company’s AI-First strategy and driving innovation through the development of strategic, AI-centric service offerings.
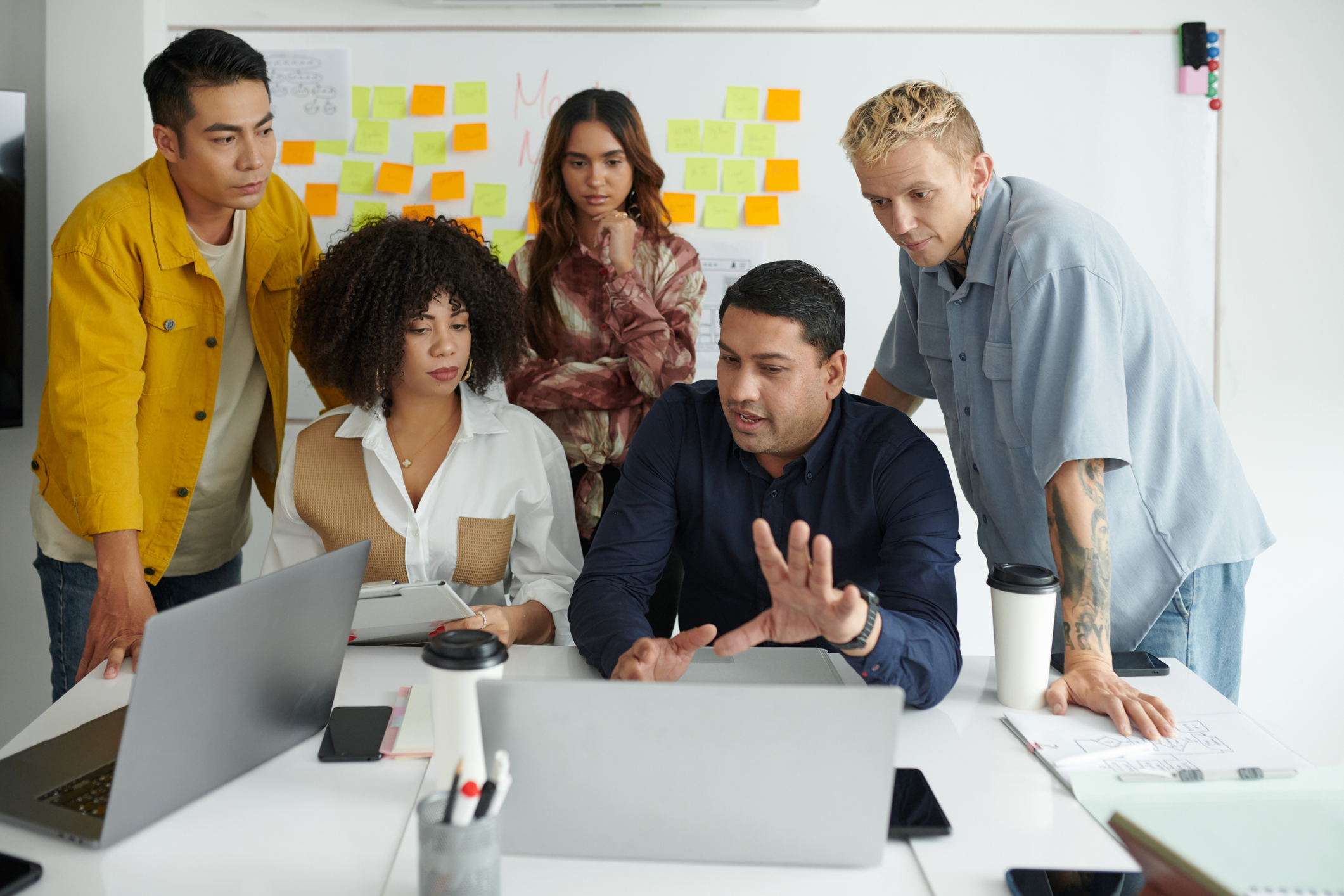
Don’t Take Product Out of the Equation: How to Nail Your AI Implementation
AI isn't just about the technology, it's about solving real problems and delivering real value. One way to do that is to keep product at the forefront during your AI implementation. Learn more about why having a product-first mindset is so important in this article by Principal Product Strategist Heather Harris.
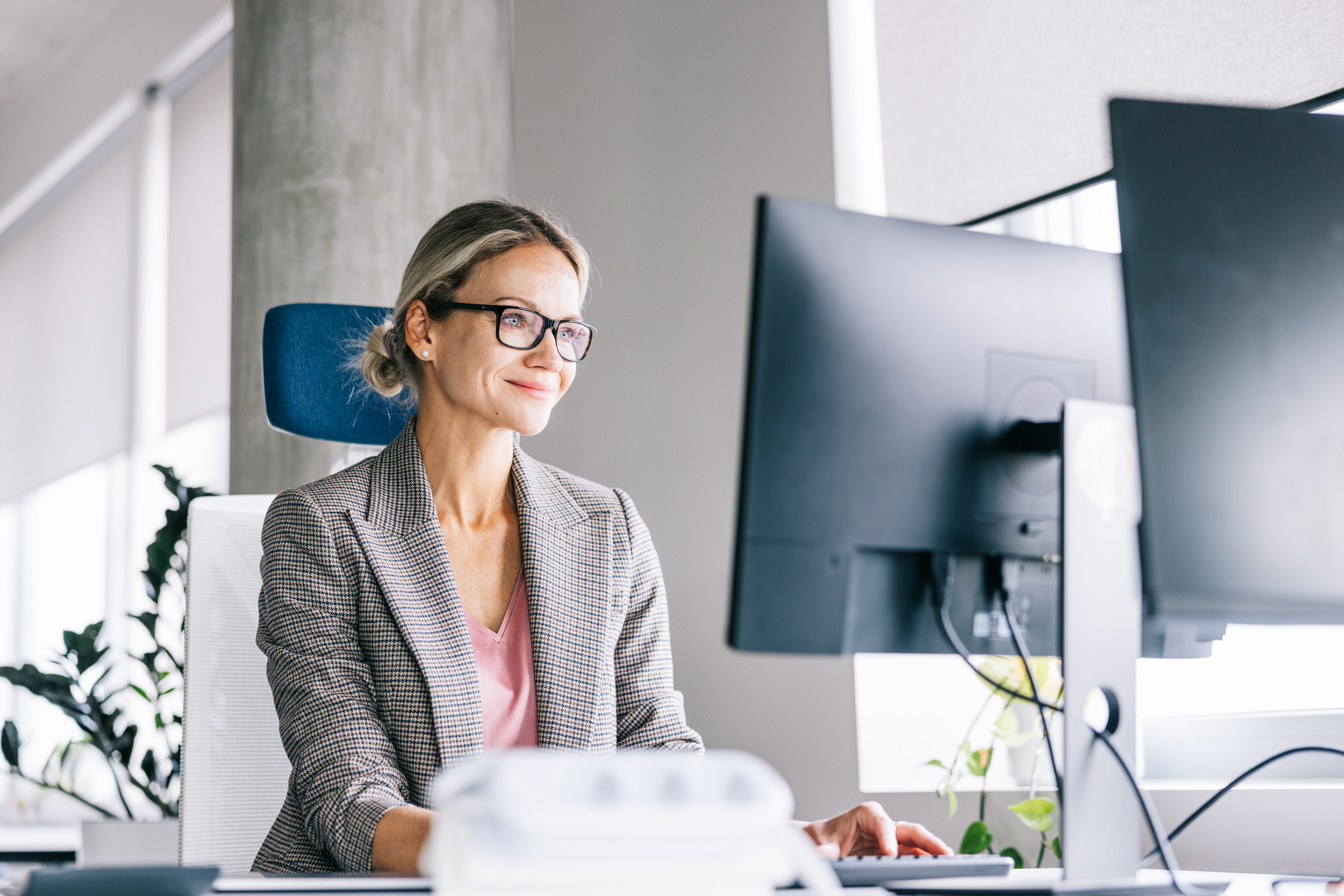
Navigating AI in Banking and Financial Services: A Risk-Based Rebellion for Leaders
Every shiny AI use case in regulated industries has a shadow: governance, compliance, model risk, ethics, bias, explainability, cyberattack vectors and more. It's not that organizations and leaders don’t want AI, it’s that they’re paralyzed by the political, regulatory, and operational realities of deploying it. Sparq's Chief Technology Officer Derek Perry and VP, BFSI Industry Leader Rob Murray argue we need to change that. Check out this article to learn how to actually ship production AI use cases in regulated environments.
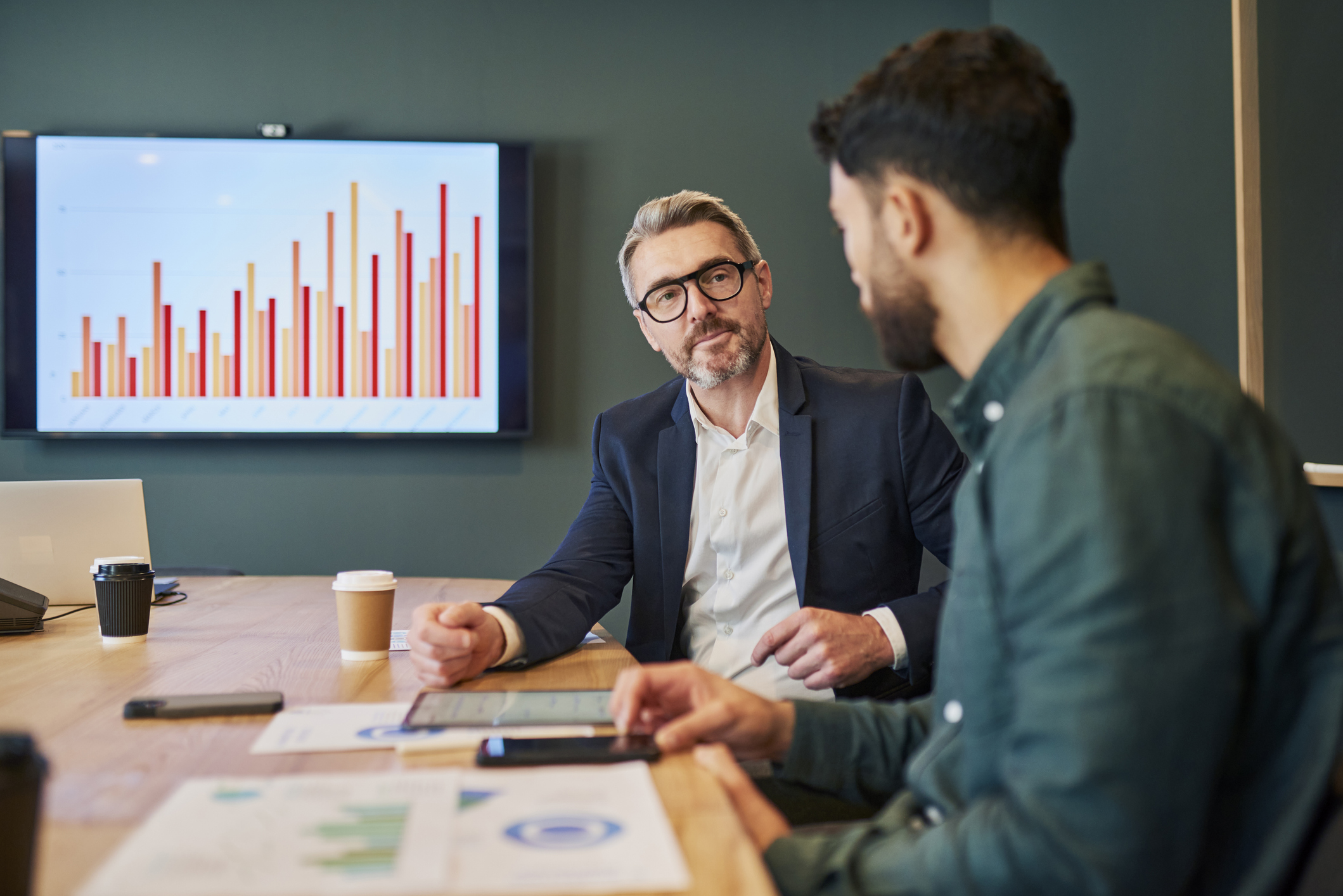
Five Important Questions to Ask Before Your AI Implementation
Creating a lasting impact with AI requires more than just technical output. In this article by Principal Product Strategist Heather Harris, learn five questions to ask before starting an AI implementation so it can deliver long-term business value.
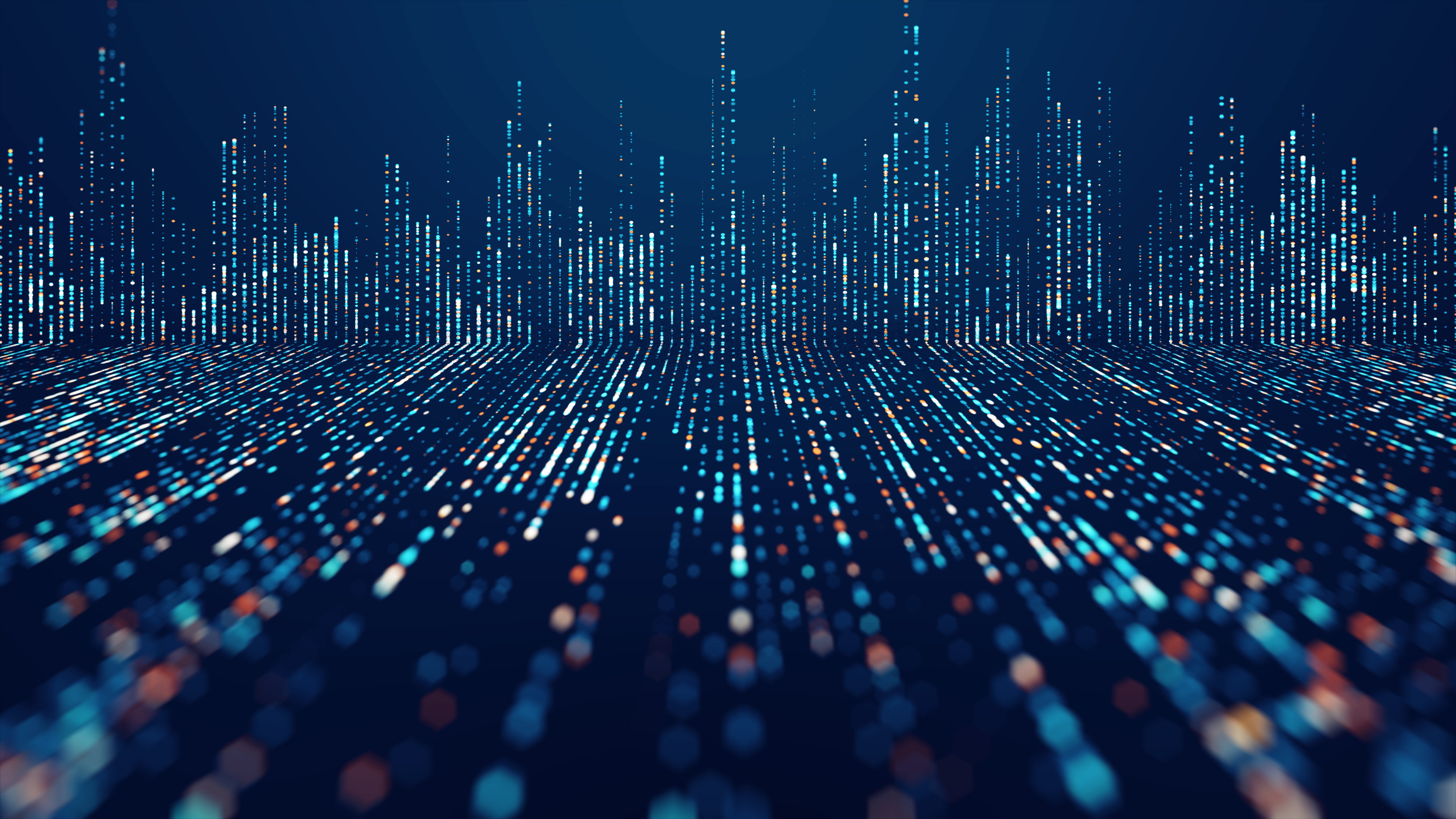
Progress Over Perfection: How to Embrace AI Now (Without Waiting for Perfect Data)
“Perfect is the enemy of good,” the old saying goes, and nowhere is that truer than in today’s race to adopt AI in business. In this article, Sparq CTO Derek Perry shares actionable steps for organizations to embrace AI now (without waiting for perfect data).